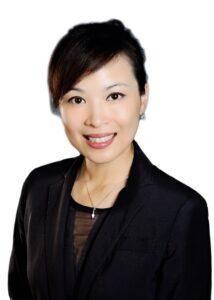
Artificial intelligence (AI) has become a central focus within various spheres, from commerce to medicine—including applications in patient care. Sharon Kiang, MD, talks to Vascular Specialist about the specter of bias and how a “hybrid” approach between AI and human specialty may be the best solution going forward.
Sharon Kiang, MD, considers it the “silver bullet” clinical perception of AI. In the Loma Linda University School of Medicine associate professor’s reckoning, it’s no mere nebulous metaphor but the underpinnings of her latest research into disease prediction models, and the gendered biases they found within their foundational algorithms.
“Originally, we weren’t looking at bias. We were just looking to see if the aortic model, using machine learning and AI algorithms, could predict and understand the progression of disease and, if possible, therefore predict outcomes,” Kiang tells Vascular Specialist. Operating on the assertion that human error and a “human-bound limitation of resources” confound patient care today, Kiang’s team believe that AI could alleviate a portion of manual “yearly factors,” which can take up significant time.
Kiang has not long since finished presenting some of her latest findings at the 2023 Women’s Vascular Summit (April 28–29) in Buffalo, New York, where she extrapolated on the advent of AI’s more frequent deployment, and the greater ease with which its limitations can be seen. “We started reading about how bias is unintentionally put into algorithms, and so went to look at our own data to see if there are biases there,” she says. Kiang selected gender as their specified bias variable, in part because disparities within post-intervention clinical outcomes in female aneurysm patients have already been reported. “We wanted to see if our machine was able to fix that and equalize its ability to learn,” Kiang explains.
Kiang told the Women’s Vascular Summit about how she and colleagues investigated gendered bias in convolutional neural networks, or deep learning, for abdominal aortic aneurysm (AAA). Their findings revealed that the machine was unable to overcome this bias and could not identify aneurysms in females as it could in males. “This may be that female aortas are smaller,” Kiang notes, so limiting the machine’s accuracy. Offering further explanation, she stated that their data may have also been “flawed,” creating bias in the algorithm, or that it may not accurately represent “the question being asked of the machine.”
Although she hypothesized that a perfect algorithm could theoretically be designed— blinded to race, gender, financial, and geographical disparities, so in essence a “blinded unicorn algorithm”—Kiang states that questions arise about whether this would make it a “useful” algorithm at all. Where the “balance” lies, she continues, is in a realistic AI architecture that can support these variables in a “representative” and “fair” way.
But, time is of the essence. “AI moves fast” Kiang asserts, observing that her growing body of research is developing closely alongside that of organizations with predominantly financial motives, who are keen to “keep pushing.” Apart from a brief diversion to comment on the novel and rapid development of ChatGPT—“Dude, we haven’t even discussed the ethical implications of [ChatGPT], right?”—Kiang’s viewpoint remains focused on the positive change AI can effect in the care of humans globally. “It sounds Ivory Tower-ish, but my mind is centered on the benefit to populations—not just at a specialty level, or even at a hospital level, but at a national, global level,” Kiang says.
AI does not come without limitations, Kiang makes clear; however, apprehension has most commonly been “driven by fear.” “On a superficial level people suggest that they will be out of a job, and I believe that’s unfounded,” she says, highlighting the only legitimate fear might be for support staff whose job could be performed more efficiently by AI. “I’m not here to say we should not advance technology so that people can be less efficient in the care that they provide patients. But neither am I saying we should build Skynet and drive the human race out of need,” she adds.
A “hybrid” approach between AI and human specialty may be the best solution, Kiang proposes, a “human understanding of relationships” filling the gaps where AI is most lacking. But a “critical mass” of people who believe in the positive function of AI is needed, she continues. “If you don’t have that buy-in, you will have a lopsided relationship that isn’t truly a hybrid model. In this case, you will have executive bean-counters who don’t know anything about boots-to-the-ground patient care, leaning on AI predictions. I can see that as a problem moving forward.”
Kiang describes her experience of the complex contemporary reception to AI, detailing how audiences are becoming more “receptive” to her ongoing AI research compared with previous years.
“We are hitting while the iron is hot right now, and it’s very hot,” she says. Kiang believes people are now more “open-minded, or are being forced to be.” Emphasizing the importance of “clean” messaging when presenting research on this technology, Kiang says she aims to continue demonstrating the realistic clinical applications of AI that have the potential to better patient care in the future.